A Data Scientist Becomes a CFO
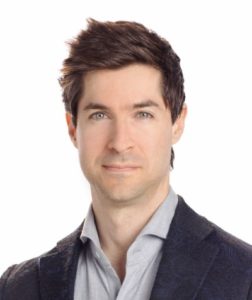
John Collins, CFO, LivePerson
John Collins likes info. As a distinctive investigator with the New York Inventory Trade, he crafted an automatic surveillance system to detect suspicious buying and selling activity. He pioneered methods for transforming 3rd-party “data exhaust” into financial investment alerts as co-founder and chief products officer of Thasos. He also served as a portfolio manager for a fund’s systematic equities buying and selling technique.
So, when making an attempt to land Collins as LivePerson’s senior vice president of quantitative technique, the software program enterprise despatched Collins a sample of the info that is produced on its automatic, synthetic intelligence-enabled discussion system. He was intrigued. Immediately after a few months as an SVP, in February 2020, Collins was named CFO.
What can a person with Collins’ form of working experience do when sitting at the intersection of all the info flowing into an running enterprise? In a cellular phone job interview, Collins mentioned the first methods he’s taken to completely transform LivePerson’s large sea of info into useful facts, why info science jobs frequently fail, and his eyesight for an AI running design.
An edited transcript of the discussion follows.
You arrived on board at LivePerson as SVP of quantitative technique. What were your first methods to modernize LivePerson’s inner functions?
The enterprise was jogging a incredibly fragmented community of siloed spreadsheets and organization software program. People executed primarily the equal of ETL [extract, completely transform, load] work opportunities — manually extracting info from one system, transforming it in a spreadsheet, and then loading it into one more system. The final result, of program, from this form of workflow is delayed time-to-action and a seriously constrained move of reliable info for deploying the simplest of automation.
The focus was to solve all those info constraints, all those connectivity constraints, by connecting some programs, writing some basic routines — largely for reconciliation purposes — and simultaneously developing a new contemporary info-lake architecture. The info lake would provide as a one source of truth of the matter for all info and the again office environment and a basis for promptly automating guide workflows.
A single of the initial areas wherever there was a major effects, and I prioritized it for the reason that of how easy it seemed to me, was the reconciliation of the funds flowing into our financial institution account to the bill we despatched shoppers. That was a guide approach that took a staff of about six men and women to reconcile bill facts and financial institution account transaction detail constantly.
More impactful was [examining] the gross sales pipeline. Traditional pipeline analytics for an organization gross sales small business is composed of taking late-phase pipeline and assuming some portion will close. We crafted what I look at to be some rather standard typical device finding out algorithms that would recognize all the [contributors] to an enhance or decrease in the probability of closing a major organization offer. If the buyer spoke with a vice president. If the buyer bought its options staff concerned. How several conferences or phone calls [the salespeson] had with the buyer. … We were then able to deploy [the algorithms] in a way that gave us perception into the bookings for [en overall] quarter on the initial day of the quarter.
If you know what your bookings will be the initial week of the quarter, and if there’s a problem, management has lots of time to program-proper right before the quarter finishes. Whereas in a typical organization gross sales scenario, the reps could keep onto all those specials they know are not likely to close. They keep onto all those late-phase specials to the incredibly end of the quarter, the previous couple of weeks, and then all of all those specials force into the up coming quarter.
LivePerson’s technological know-how, which suitable now is largely aimed at buyer messaging by your purchasers, could also have a position in finance departments. In what way?
LivePerson provides conversational AI. The central plan is that with incredibly shorter text messages coming into the system from a customer, the device can understand what that customer is interested in, what their desire or “intent” is, so that the enterprise can possibly solve it straight away by way of automation or route the issue to an suitable [buyer company] agent. That understanding of the intent of the customer is, I consider, at the reducing edge of what is achievable by way of deep finding out, which is the foundation for the form of algorithms that we’re deploying.
The plan is to apply the exact form of conversational AI layer across our programs layer and above the prime of the info-lake architecture.
You would not need to have to be a info scientist, you would not need to have to be an engineer to simply just request about some [economic or other] facts. It could be populated dynamically in a [consumer interface] that would allow for the person to investigate the info or the insights or uncover the report, for case in point, that covers their domain of curiosity. And they would do it by simply just messaging with or speaking to the system. … That would completely transform how we interact with our info so that all people, no matter of background or skillset, had obtain to it and could leverage it.
The purpose is to develop what I like to consider of as an AI running design. And this running design is based on automatic info seize — we’re connecting info across the enterprise in this way. It will allow for AI to run practically just about every program small business approach. Each approach can be broken down into scaled-down and scaled-down components.
“Unfortunately, there’s a misconception that you can retain the services of a staff of info experts and they’ll get started providing insights at scale systematically. In fact, what comes about is that info science results in being a compact group that is effective on advertisement-hoc jobs.”
And it replaces the classic organization workflows with conversational interfaces that are intuitive and dynamically built for the particular domain or problem. … Men and women can eventually cease chasing info they can get rid of the spreadsheet, the maintenance, all the glitches, and focus in its place on the resourceful and the strategic work that tends to make [their] position fascinating.
How far down that road has the enterprise traveled?
I’ll give you an case in point of wherever we’ve already sent. So we have a manufacturer-new planning system. We ripped out Hyperion and we crafted a economic planning and investigation system from scratch. It automates most of the dependencies on the price side and the earnings side, a great deal of wherever most of the dependencies are for economic planning. You really do not discuss to it with your voice yet, but you get started to kind a little something and it acknowledges and predicts how you will finish that lookup [query] or plan. And then it auto-populates the specific line merchandise that you might be interested in, offered what you have typed into the system.
And suitable now, it is far more hybrid reside lookup and messaging. So the system eradicates all of the filtering and drag-and-fall [the consumer] had to do, the infinite menus that are typical of most organization programs. It truly optimizes the workflow when a person wants to drill into a little something that’s not automatic.
Can a CFO who is far more classically experienced and does not have a background have in info science do the forms of things you are carrying out by selecting info experts?
Unfortunately, there’s a misconception that you can retain the services of a staff of info experts and they’ll get started providing insights at scale systematically. In fact, what comes about is that info science results in being a compact group that is effective on advertisement-hoc jobs. It creates fascinating insights but in an unscalable way, and it just cannot be used on a normal foundation, embedded in any form of real determination-earning approach. It results in being window-dressing if you really do not have the suitable talent established or working experience to control info science at scale and ensure that you have the proper processing [abilities].
In addition, real experts need to have to work on difficulties that are stakeholder-pushed, devote fifty% to eighty% of their time not writing code sitting in a dark place by by themselves. … [They are] speaking with stakeholders, understanding small business difficulties, and ensuring [all those discussions] form and prioritize almost everything that they do.
There are info constraints. Data constraints are pernicious they will cease you cold. If you just cannot uncover the info or the info is not linked, or it is not conveniently available, or it is not clean, that will quickly just take what might have been hours or days of code-writing and switch it into a months-long if not a year-long challenge.
You need to have the proper engineering, precisely info engineering, to ensure that info pipelines are crafted, the info is clean and scalable. You also need to have an efficient architecture from which the info can be queried by the experts so jobs can be run promptly, so they can take a look at and fail and master promptly. Which is an important aspect of the general workflow.
And then, of program, you need to have again-end and front-end engineers to deploy the insights that are gleaned from these jobs, to ensure that all those can be generation-amount high quality, and can be of recurring price to the procedures that drive determination earning, not just on a one-off foundation.
So that full chain is not a little something that most men and women, specially at the optimum amount, the CFO amount, have had an prospect to see, permit by itself [control]. And if you just retain the services of somebody to run it without the need of [them] possessing had any initial-hand working experience, I consider you run the chance of just form of throwing things in a black box and hoping for the greatest.
There are some rather serious pitfalls when dealing with info. And a popular one is drawing most likely defective conclusions from so-known as compact info, wherever you have just a couple of info points. You latch on to that, and you make conclusions appropriately. It’s truly easy to do that and easy to forget the fundamental statistics that enable to and are essential to attract truly valid conclusions.
Without the need of that grounding in info science, without the need of that working experience, you are lacking a little something rather essential for crafting the eyesight, for steering the staff, for placing the roadmap, and in the long run, even for executing.